Data, Software and Talent: Turning Open Data into a useful Anti-Corruption Tool for Africa
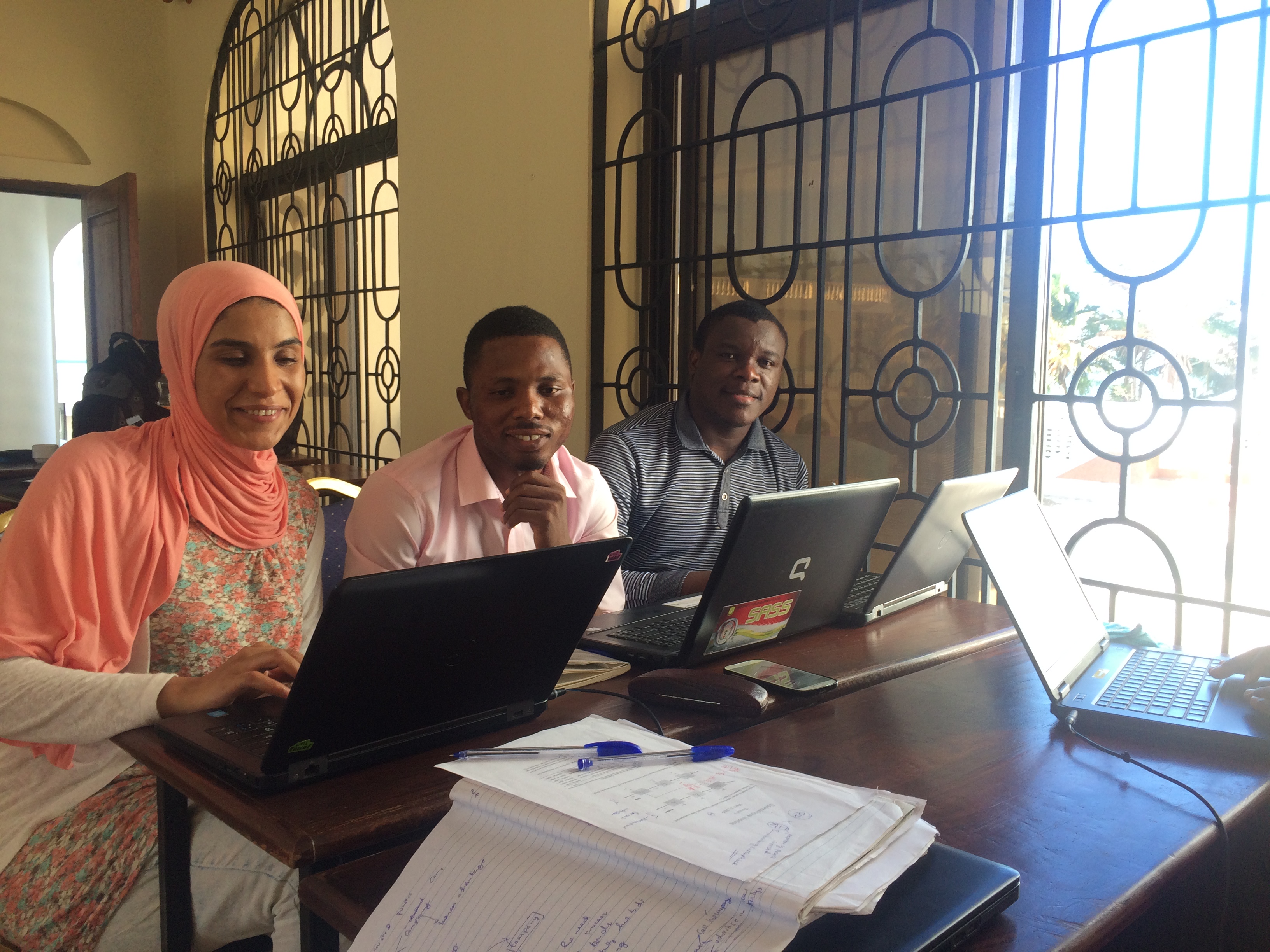
For years, campaigners have lauded the benefits of transparency as a policy solution to corruption. That message was gradually refined as researchers noted that transparency was effective as an anti-corruption tool only if it led to increased accountability, and that this only happened in conditions where the overall institutional environment was conducive.
More recently, open data – data about the activities of governments and public officials which can shine light on whether they perform their duties with integrity – has again been paraded as the variant of transparency that will make all the difference.
Again, it quickly became clear that open data only helps detect and deter graft in certain conditions: first, the data must be good quality, second, there must be a group of interested and informed users, and third, there must be a way of getting the results heard, investigated, and acted upon. If open data is to work as a global anti-corruption tool, that implies a major effort to improve the quality of data that governments publish, empower actors to use the data to uncover misconduct, and then lobby governments and donors to listen to and act on the findings.
In the last few months we have been involved in a project, led by Oxford mathematician Balázs Szendrői, that helps empower an important but often overlooked set of potential users – African mathematicians, whose statistical analysis skills are key to forming an evidence base for future public policy-making. The project collected new procurement data, developed a new software tool for analysis and trained the maths students in how to look for corruption indicators.
The data concern how aid money is spent through national procurement systems. As part of our British Academy/DFID-funded project, we (Liz Dávid-Barrett, Mihály Fazekas and Olli Hellmann) a have collected contract-level data from three major donors. This was a lengthy exercise, involving scraping data from a number of disparate documents and files, checking for missing data and resolving numerous irregularities. Yet we now have a dataset comprising more than half a million contracts and stretching back almost 20 years.
The tools are analytical and statistical. We utilise a method developed by Mihály to identify ‘red flags’ in the data which might be indicative of corruption risks in the procurement process – that is, ways in which a supposedly open competition can be manipulated to favour a certain company. However, using the method to analyse data requires statistical skills and software. Both are in short supply in Africa – mathematicians have not traditionally received a very strong training in statistical methods, and cash-strapped universities have generally lacked the resources to fund and update licences to software packages that facilitate such analysis.
The African Maths Initiative(AMI), a Kenyan NGO that works to create a stronger mathematical community and culture of mathematics across Africa, has helped to solve this problem by developing a new open-source software for statistical analysis. Based on the widely used programme, R, their package provides a ‘front end’ that makes R much more user-friendly and accessible. The software, R-Instat, has been funded through crowd-sourcing and developed in cooperation with African mathematicians. Still in development, it is on track for launch in July this year. In the meantime, we have worked with AMI to develop a menu on R-Instat that can be used specifically for analysing procurement data and identifying corruption risk indicators.
So, we have the data, we have the tools, what about the skills? For data to be useful as an anti-corruption tool, we need to bring together two groups: people who understand how to analyse data, and people who understand how procurement systems can be manipulated to corrupt ends – the latter is where I came in. In March I visitedAIMS Tanzania, an institute that offers a one-year high-level Master’s programme to some of Africa’s best maths students. In a one-day workshop, I spent the morning teaching the maths students about how academics study corruption. We took our time with getting definitions right to make sure everyone was on the same page, and then launched into the ways in which the procurement process can be corrupted and how that might manifest in certain red flags, such as there being only one bidder for a contract. The students were a great pleasure to work with – highly engaged and posing thoughtful questions that related to their own experiences of corruption.
But the real excitement came in the afternoon when we tried out R-Instat. Students formed teams while David Stern and Danny Parsons, two of the software’s developers, asked them to develop research questions that could be investigated using our World Bank dataset. They could then use R-Instat to run analyses on the data and find answers to their questions. I was on hand to answer corruption-specific queries and suggest what else to look for, while Danny and the R-Instat team members helped them navigate the software.
This was a corruption nerd’s idea of paradise. Even the simplest analyses revealed interesting patterns in the data. Why did one country’s receipts from the World Bank drop off a cliff one year and never recover? Discussion revealed a few possible reasons: perhaps a change of government led donors to change policy, or the country reached a stage of development where it no longer qualified for aid? The students became more and more motivated as they realised how statistical methods could be applied to identify, understand and solve real-world problems that had seemed intractable and inevitable.
Some of the teams came up with really provocative questions, such as the group who wanted to know whether Francophone or Anglophone countries were more vulnerable to corruption risks. Their initial analysis revealed that contracting in the Francophone countries was more associated with red flags. They developed the analysis to include a wider selection of countries, and maintained broadly similar results. With a little more time, we could have introduced controls for development levels and other factors.
Another group found that one-quarter of contracts let in the education sector in one country had been won by just one company. We investigated further: it was important to look at the value of contracts too, not just the number. This analysis revealed a yet more worrying result: more than half of total contract value in this sector had been won by three companies, all of which had suspiciously similar names. Again, there might be perfectly innocent reasons for this, but in just a couple of hours, we had a set of preliminary results that pointed up a number of directions for future research. Imagine what we might find with a little more time!